Y2 you的問題,我們搜遍了碩博士論文和台灣出版的書籍,推薦unknow寫的 你的歌我來唱(4)──當代中文藝術歌曲集 可以從中找到所需的評價。
另外網站Line segments and curves — geom_segment • ggplot2也說明:You must supply mapping if there is no plot mapping. ... y1 = 21.0, y2 = 15.0) b + geom_curve(aes(x = x1, y = y1, xend = x2, yend = y2, colour = "curve"), ...
國立中央大學 產業經濟研究所 鄭有為所指導 蔡佳儒的 論破產撤銷權─以美國聯邦破產法典作比較 (2021),提出Y2 you關鍵因素是什麼,來自於破產撤銷權、偏頗行為、詐害行為、詐欺性移轉、消費者債務清理條例、債務清理法草案。
而第二篇論文亞洲大學 資訊工程學系 薛榮銀所指導 Prayitno的 使用聯盟式學習於跨機構非獨立同分布醫療資料預測模型之開發 (2021),提出因為有 Federated Learning、melanoma、non-IID、skin lesion image classification的重點而找出了 Y2 you的解答。
最後網站3.2 Higher Order Partial Derivatives則補充:You are familiar with the chain rule for functions of one variable: if f is ... u = x2y v = 3x + 2y. 1. Find ∂2z. ∂y2 . Solution: We will first find.
你的歌我來唱(4)──當代中文藝術歌曲集
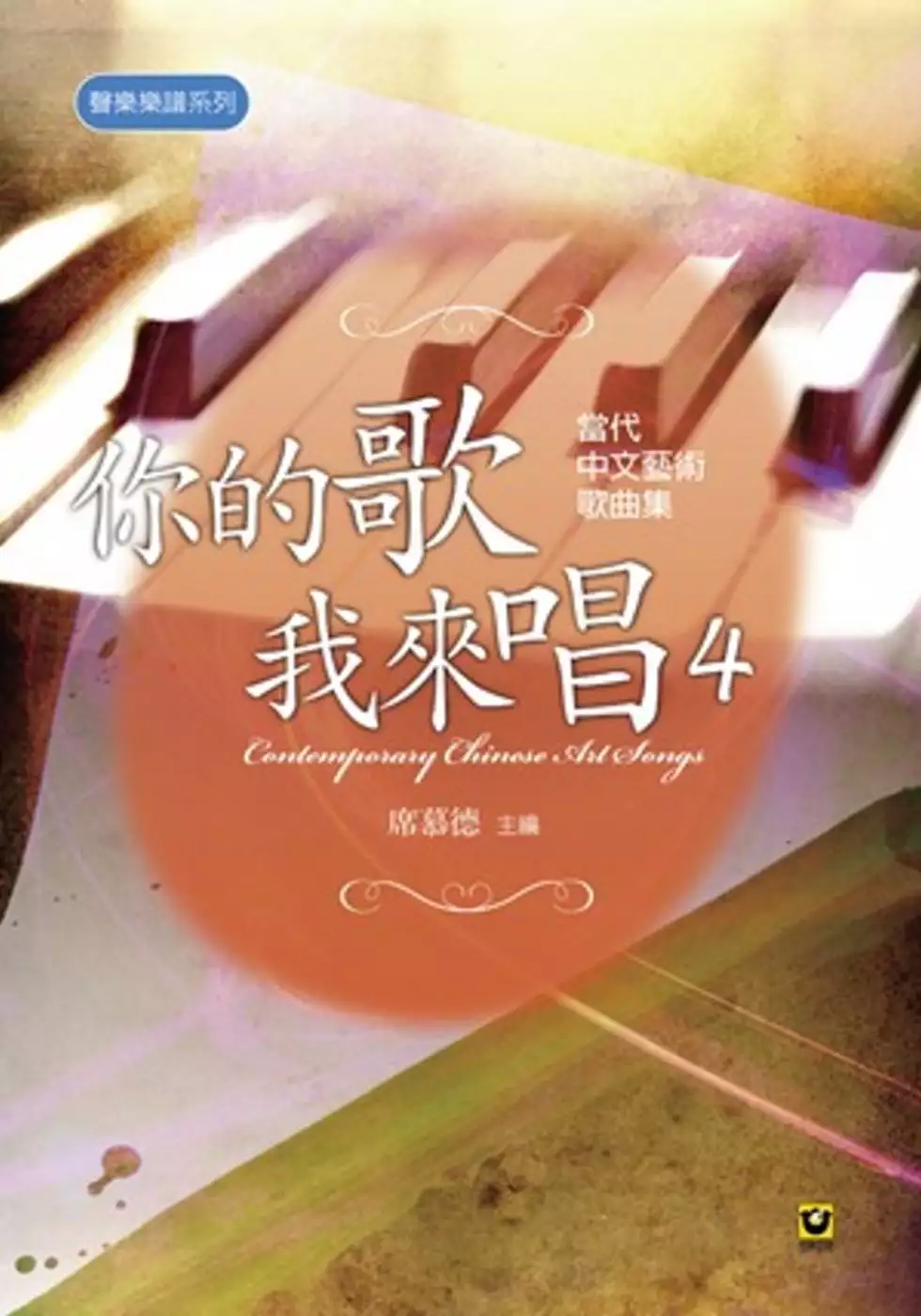
為了解決Y2 you 的問題,作者unknow 這樣論述:
聲樂重唱是多聲部歌曲的一種演唱形式,常應用在歌劇、音樂劇或重唱音樂會中,而中文重唱歌曲的數量,與西方相比可說是鳳毛麟角。《你的歌我來唱》第四集,即是「聲樂家協會」為關懷國家文化發展謹盡棉薄的又一次成果及見證。 這本歌集的特色,在於「以四位女詩人的詩作譜寫重唱曲」,堪稱樂界創舉。由陳茂萱、鍾耀光、李子聲、陳瓊瑜為林婉瑜、陳育虹、席慕蓉、零雨的詩作譜寫的新曲,包括二重唱、三重唱與四重唱,聲部則含括女高音、次女高音及男高音,不僅形式多樣,且風格各異,如悲悽、荒涼,或活潑、俏皮,亦有節奏快速和寬廣豪放者;期盼您細細品味這些屬於我們的歌。
Y2 you進入發燒排行的影片
choreographed
by akanenmiyoshi
Hope you like it!
booking: [email protected]
Instagram: @akanenmiyoshi
**NO COPYRIGHT INFRINGEMENT INTENDED***
"This video uses copyrighted material in a manner
that does not require approval of the copyright
holder. It is a fair use under copyright law.
"quotation of excerpts in a review or criticism for
purposes of illustration or comment; quotation of
short passages in a scholarly or technical work,
for illustration or clarification of the author's
observations; use in a parody of some of the
content of the work parodied; summary of an
address or article, with brief quotations, in a news
report; reproduction by a library of a portion of a
work to replace part of a damaged copy;
reproduction by a teacher or student of a small
part of a work to illustrate a lesson; reproduction
of a work in legislative or judicial proceedings or
reports; incidental and fortuitous reproduction, in
a newsreel or broadcast, of a work located in
論破產撤銷權─以美國聯邦破產法典作比較
為了解決Y2 you 的問題,作者蔡佳儒 這樣論述:
破產撤銷權得以重新檢視債務人於破產程序開始前實施之財產處分行為,並將已歸於消滅之債權債務關係予以撤銷,乃破產法上實現債權人平等之一大關鍵。我國破產撤銷權制度自民國二十四年破產法實施時起即已存在,至今未進行重大修法改革,其作為防止債務人財產不當減損以及保障全體債權人公平受償之重要制度,實有重新檢視並加以修正之必要,因此本文對我國破產撤銷權制度加以探討,並透過比較法學之方式,以破產法學發展蓬勃之美國聯邦破產法典作為我國借鑒,分析我國破產撤銷權制度有何不足之處,進而提出本文看法以及相關修法建議。
使用聯盟式學習於跨機構非獨立同分布醫療資料預測模型之開發
為了解決Y2 you 的問題,作者Prayitno 這樣論述:
Artificial intelligence (AI) development has attracted significant attention for skin lesion prediction with data-driven insight to assist dermatologists' diagnosis and improve patients' quality of care. A diverse and considerable number of medical data are required from cross-institutional data si
los to develop a robust AI model. Unfortunately, because these massive numbers of medical data are challenging to collect, privacy-sensitive, and prone to single institutional bias, they cannot be trained in a centralized manner. The federated learning (FL) method provides cross-institutional AI mod
el development without using a centralized data aggregated server for medical data transmission to address privacy and institutional bias concerns. However, some studies show that the performance drops significantly with the non-identically and independently distributed (non-IID) data distribution s
cenario. Additionally, from the clinical practitioner's viewpoint, it is hard to interpret the prediction results from the AI models. This study presents a robust end-to-end framework in skin lesion classification tasks with FL, non-IID, and interpretability strategies to address the earlier challen
ges. In particular, data availability and privacy-preserving to develop a robust AI model can be achieved using the FL strategy. Meanwhile, considering the systemic differences in skin lesions data distributions from different sites, the FL model is optimized with the non-IID degree strategy to impr
ove the model performance. Additionally, interpretability of the classification results can be presented using an explainable artificial intelligence (XAI) module to support dermatologists in making fast and reliable diagnostics. The experimental results show that the FL scheme increases the AUROC s
cores of the ANN, CNN, DenseNet, EfficientNet, ResNet, and VGG by 24.2%, 16.6%, 2%, 6.1%, 5.1%, and 2.3 % on average respectively than training the models in the single-institution scheme. Furthermore, the average AUROC score performed by the proposed weighting coefficient is 8.9% and 3%, higher tha
n those achieved by the simple FedAvg, and FedAvg, respectively.
想知道Y2 you更多一定要看下面主題
Y2 you的網路口碑排行榜
-
#1.y2 , is that you (@y2tnb) / Twitter
ns+ ultra . out now . neverever , louvre , & blitz all prod clayco bryce & myself . Image. 7. 8. 127. Show this thread · y2 , is that you Retweeted. 於 twitter.com -
#2.Use of the TI-84 in Math 142
Enter the left hand side of the equation in Y1 and the right hand side of the equation in Y2. Choose an appropriate window and graph both equations so you ... 於 www.math.tamu.edu -
#3.Line segments and curves — geom_segment • ggplot2
You must supply mapping if there is no plot mapping. ... y1 = 21.0, y2 = 15.0) b + geom_curve(aes(x = x1, y = y1, xend = x2, yend = y2, colour = "curve"), ... 於 ggplot2.tidyverse.org -
#4.3.2 Higher Order Partial Derivatives
You are familiar with the chain rule for functions of one variable: if f is ... u = x2y v = 3x + 2y. 1. Find ∂2z. ∂y2 . Solution: We will first find. 於 www.ucl.ac.uk -
#5.you² | Breakthrough Performance Results | Book | Pritchett LP
This is the power of “you squared” . . . the quantum leap strategy for breakthrough performance. In you², Price Pritchett outlines a unique, dynamic method for ... 於 www.pritchettyou2.com -
#6.Implicit Differentiation - Mathcentre
In order to master the techniques explained here it is vital that you undertake ... In order to differentiate y2 with respect to x we have differentiated. 於 www.mathcentre.ac.uk -
#7.從YouTube下載視頻和音頻 - Y2mate.com
Download video, mp3 from youtube for PC, mobile, android, ios free. Support downloading all Video formats: Mp4, Mp3, 3GP, webm, hd videos from youtube. 於 www.y2mate.com -
#8.Two Stage Least Squares
cov(z1,u) = 0, cov(y2,u) = 0 y2 is endogenous: OLS estimation gives inconsistent estimates because y2 is correlated with u we need an instrument, say z2, ... 於 www.eco.uc3m.es -
#9.How to Wire a Thermostat - Honeywell Home
Y2 – Compressor Stage 2 (Cooling) G – Fan C – Common U – Humidifier, Dehumidifier, or Ventilator control. L/A – A – Input for heat pump ... 於 www.honeywellhome.com -
#10.Think You Live - lagu dan lirik oleh Y2 - Spotify
Think You Live · Track Populer oleh Y2 · Rilis Populer oleh Y2 · Album Populer oleh Y2 · Single dan EP Populer oleh Y2. 於 open.spotify.com -
#11.If (x + iy)^3 = u + iv , then prove that u/x + v/y = 4(x^2 - Toppr
Click here to get an answer to your question ✍️ If (x + iy)^3 = u + iv , then prove that u/x + v/y = 4(x^2 - y^2) . ... x3+i3y3+3x2yi+3xy2i2=u+iv 於 www.toppr.com -
#12.A visit to a synagogue - KS1 Religious Education - BBC
Ella is stood next to the Ner Tamid. Watch: Meet Ella as she takes you on a tour around her local synagogue. 於 www.bbc.co.uk -
#13.Y2 Rap | By Valley View Primary School | Facebook - Facebook
Y2 have written and performed a rap for you to show just how kind our Valley View children are. We hope you enjoy their cool moves! 於 www.facebook.com -
#14.YtMp3 - YouTube to MP3 Converter
Our Web-App YTMP3 allows you to download your favorite YouTube videos as MP3 (audio) or MP4 (video) files in the most efficient way. You are able to use our ... 於 ytmp3.nu -
#15.Y2 - "It's My World" (Official Audio) - YouTube
0:00 · New! Watch ads now so you can enjoy fewer interruptions. Got it ... 於 www.youtube.com -
#16.Livato Y2 WiFi Projector with Built-in YouTube ... - JioMart
This is a high quality Livato portable home theater projector that you can watch movies, sports shows, movies, photos, etc. For example, if you need to ... 於 www.jiomart.com -
#17.Rural Access to Primary and Preventive Services (RAPPS ...
CMS approved RAPPS for SFY 2023 (Y2) on August 1, 2022. ... If you are participating in the RAPPS program, you will use the reporting portal to access ... 於 www.hhs.texas.gov -
#18.SOLUTIONS TO HOMEWORK ASSIGNMENT #4, MATH 253
It is now clear that. ∂u. ∂t. = ∂2u. ∂x2. +. ∂2u. ∂y2 . 3. (a) Find an equation of the tangent plane to the surface x2 + y2 + z2 = 9 at the point. ( ... 於 personal.math.ubc.ca -
#19.Finding Intersections
When selecting the two curves to use, the TI-82 defaults to y1 and y2, so if those are the curves you're using, you just hit enter when it asks for the ... 於 people.richland.edu -
#20.Misc 16 - If (x + iy)3 = u + iv, then show that u/x + v/y - Teachoo
Misc, 16 If (x + iy)3 = u + iv, then show that u/x + v/y = 4 (x2 – y2) . We know that (a + b)^3 = a3 + b3 +3ab (a + b) Replacing a ... 於 www.teachoo.com -
#21.Conic Sections and Standard Forms of Equations
If you divide both sides of the first equation x2+4y2=16 by 16 you get x216+y24=1 . That is, it is an ellipse centered at origin with major axis 4 and minor ... 於 www.varsitytutors.com -
#22.Slope - Kids Math - Ducksters
If you pick two points on a line --- (x1,y1) and (x2,y2) --- you can calculate the slope by dividing y2 - y1 over x2 - x1. 於 www.ducksters.com -
#23.To-y2 專輯歌曲Kis-My-Ft2 ※ Mojim.com 魔鏡歌詞網
... 歌詞りあらぶ歌詞HANDS UP歌詞Cannonball歌詞Letting Go歌詞Positive Man歌詞MAHARAJA歌詞Be alright歌詞My Place歌詞Edge of Days歌詞Make you mine歌詞Sometime. 於 mojim.com -
#24.Y2 - YouTube
By way of Austin, TX, Y2 lives up to his city's slogan, "Keep Austin Weird" thriving far outside of any perceived genre or creative comfort zone. 於 www.youtube.com -
#25.C Program: Calculate the distance between the two points
Input x1: 25 Input y1: 15 Input x2: 35 Input y2: 10 Distance ... You can use itoa() function to convert your integer value to a string. 於 www.w3resource.com -
#26.What do my thermostat's wire labels mean? - ecobee Support
If you have a two-stage compressor, Y2 controls your compressor's second stage, allowing for different levels of heating and cooling. 於 support.ecobee.com -
#27.Use the keyboard to work with the ribbon in Word
When you press Alt, letter or KeyTips appear on the ribbon by tabs or Quick Access buttons. ... Alt+Y2 or F1 and then enter the search term. 於 support.microsoft.com -
#28.YU2 Powered Desktop Speakers - Kanto Audio
YU2 Powered Desktop Speakers feature a built-in soundcard, letting you stream high-quality audio directly from your computer's USB port. 於 www.kantoaudio.com -
#29.Speech Marks Game. 7 (42 reviews) PlanIt Y4 SPaG Lesson ...
763 Top "Speech Marks Worksheets" Teaching Resources curated for you. Y2 Y3. Speech Punctuation Game Subject: English Age range: 7-11 Resource type: ... 於 9411.ru -
#30.Distance On A Coordinate Plane Calculator. The formula for ...
This tool will help you calculate the distance between two coordinates or a ... Analytic geometry >. d = √ (x2 - x1)2 + (y2 - y1)2 + (z2 - z1)2. . a^2 + ... 於 vgoryshop.ru -
#31.MATLAB plotyy - MathWorks
plotyy(X1,Y1,X2,Y2) plots Y1 versus X1 with y-axis labeling on the left and ... Function handles enable you to access user-defined local functions and can ... 於 www.mathworks.com -
#32.6.2.4 Cauchy Schwarz Inequality
For any two random variables X and Y, we have |EXY|≤√E[X2]E[Y2],. where equality holds if and only if X=αY, for some constant α∈R. You can prove the ... 於 www.probabilitycourse.com -
#33.Identify a thermostat wire - Google Nest Help
You 'll need to check your current thermostat's wires to tell if your system is Nest thermostat compatible, and which thermostat models it will work with. 於 support.google.com -
#34.College Algebra with Applications for Business and Life Sciences
For instance, consider the two points (x1, y1) and (x2, y2) on the line shown in Figure 2.21. As you move from left to right along this line, a change of y2 ... 於 books.google.com.tw -
#35.Y2mate: Youtube Downloader - Download Youtube Video ...
By using our converter you can easily convert YouTube videos to mp3 (audio) or mp4 (video) files and download them for free.(y2 mate|y2mate com|ymate|y2meta). 於 y2mate.tools -
#36.How do you factor x2 y2? Maths Q&A - Byju's
How do you factor x2 y2? Get the answer to this question and access a vast question bank that is tailored for students. 於 byjus.com -
#37.y2 - SVG: Scalable Vector Graphics - MDN Web Docs - Mozilla
The y2 attribute is used to specify the second y-coordinate for drawing an ... You can use this attribute with the following SVG elements:. 於 developer.mozilla.org -
#38.Subcolumn titles - GraphPad Prism 9 User Guide
You 'll see the subcolumn tab of the Format Data Table dialog. Shortcut: Double click on any subcolumn tiltle (A:Y1 or B:Y2..). subcolumntitlesdialog. If ... 於 www.graphpad.com -
#39.Tangent Lines - Sage Calculus Tutorial - SageMath
will give you the slope between points (x1, y1) and (x2, y2). So if, for instance, we were to calculate the slope between two points on f(x) = x 3 /2, say ( ... 於 www.sagemath.org -
#40.draw_rectangle
With this function you can draw either an outline of a rectangle or a filled rectangle where the (x1,y1) position is the top left corner and the (x2,y2) ... 於 manual.yoyogames.com -
#41.電腦王阿達y2meta 下載Youtube 影片超方便,只要一個網址搞定!
接下來就會看到下方有「Video」、「MP3」、「Audio」三種選項可以選擇下載,而影片的部分可以直接下載1080p 60 的MP4 格式,真的很不錯。 於 www.kocpc.com.tw -
#42.Lecture 5 Vector Operators: Grad, Div and Curl
The mechanics of taking the grad, div or curl, for which you will need to brush ... But r = √x2 + y2 + z2, so ∂r/∂x = x/r and similarly for y,z. ⇒ ∇U =. 於 www.lehman.edu -
#43.Algebra 2 Semester 1 Final Exam Cheat Sheet. A) y = 5 1 x + 80
If you complete and understand this review packet Semester 1 Final Exam Review Video ... of Cubes x 3−y= (x−y) x2+ xy+ y2 Sum of Cubes x3 . 於 nika-hotel.ru -
#44.If you are solving y2 + 2y = 48 by completing the square, the ...
If you are solving y2 + 2y = 48 by completing the square, the next line would be If you are ... We have to find the next step while solving y2 + 2y = 48. 於 www.cuemath.com -
#45.The Substitution Method
In the substitution method you solve for one variable, ... Equation B gives you the value of y, y = 2, so you can substitute 2 into Equation A for y. 於 content.nroc.org -
#46.下載Youtube影片| Mydowndown(買噹噹)影片下載工具
至本站https://mydowndown.com/y2 輸入您取得的Youtube網址. 3. 按下『取得影片』連結按鈕即可獲得影片下載連結. 注意事項. 1. 本站支援以下 Youtube網址格式(範例). 於 mydowndown.com -
#47.How To Calculate the Average Rate of Change in 5 Steps
If you are working with two sets of coordinates, you can use this formula to find the average rate of change: (y1-y2) / (x1-x2). 於 www.indeed.com -
#48.Redmi Y2 - 16MP AI - Selfie @₹8,999 - Mi India
What makes Redmi Y2 so unique? It's a smartphone designed for the youth , and comes with a selfie camera designed to capture you at your best. 於 www.mi.com -
#49.Understanding the Dual Y Axis in Charts - Oracle Help Center
... you can plot one or more data series on a secondary vertical (Y) axis. ... screenshot showing a chart with the Y1 axis on the left and a Y2 axis on. 於 docs.oracle.com -
#50.Directional Derivatives
derivative at (a,b) in the direction of u. u = uxi + uyj and. Duf(a,b) = u·∇f(a,b). ... EX 2 For z = f(x,y) = x2 + y2, interpret gradient vector. ∇f(a,b). 於 www.math.utah.edu -
#51.Completing the Square
Leave blanks on each side of the equation for values you will add in the next step. ... If needed, divide to make the coefficients x2 and y2. 於 www.lsco.edu -
#52.Quadric Surfaces
Circle x2 + y2 = 3. (b) Sketch all the traces that you found in part (a) on the same coordinate axes. (c) Compute equations for the traces in the y = 0, ... 於 www.math.drexel.edu -
#53.3x 5y 2
How do you find the slope of the graph of 3x+ 5y = 10 ? ... Pre-Algebra Find the Slope 3x+5y-2=0 3x + 5y − 2 = 0 3 x + 5 y - 2 = 0 Rewrite in ... 於 business-online-verzeichnis.de -
#54.Phonics screening check: administration guidance - GOV.UK
If you cannot establish whether the pupil took the check in the previous year, the pupil should take the check. 4.1 Pupils absent during check week. If a pupil ... 於 assets.publishing.service.gov.uk -
#55.10-701 Machine Learning: Assignment 1
You have just got a loaded 6-sided dice from your statistician friend. ... E[(f(X) − E[Y |X])2] + E[(E[Y |X] − Y )2]+2E[(f(X) − E[Y |X])(E[Y |X] − Y )]. 於 www.cs.cmu.edu -
#56.Mathematical Statistics with Applications - 第 314 頁 - Google 圖書結果
If it is not, the method of transformations cannot be used, and you should ... Letting g(y1 ,u) denote the joint density of Y1 and U, we have, with y2 = u ... 於 books.google.com.tw -
#57.Coaching - Psychologues Consultants Y2
At Y2CP our coaches will assist you in assessing, developing and/or deepening your core competencies whether you are an executive in need of executive ... 於 y2cp.com -
#58.Worked example: slope from two points (video) - Khan Academy
When finding slope of any 2 ordered pairs, for instance lets just use (2,9) and (19,10), a simple and quick method you can use is y2 -y1 over x2-x1. 於 www.khanacademy.org -
#59.Locations near you - Y2academy
Online Vitual Class at Y2 Academy Main Office. Click 'Registration & Payment' on the front page. Then, select 'Cherry Hill (NJ)' and fill out the form. 於 www.y2academy.com -
#60.Y2 - "My Way (Can't Stop Me Now)" (Official Audio) - YouTube
0:00 · New! Watch ads now so you can enjoy fewer interruptions. Got it ... 於 www.youtube.com -
#61.YUV - 维基百科,自由的百科全书
Y'UV422编辑. Input:讀取Y'UV的4bytes(u, y1, v, y2): Output:寫入RGB的6bytes ... 於 zh.wikipedia.org -
#62.Y2 / 歪兔WaiTu ch. - YouTube
抱歉~讓各位久等了,這是拖了很久的COVER (雖然動畫很早就做完了) 歪兔自己不太會唱歌及混音,唱的不好聽還請見諒!!! 於 www.youtube.com -
#63.How do you find dy/ dx for 4 + xy = y^2? - Socratic
Use implicit differentiation with the product and power rules. Explanation: Because of the topic under which this was posted (and because ... 於 socratic.org -
#64.The curve y2 = ux3 + v passes through the point P(2, 3) and ...
Attention CA Foundation Students! To make sure you are not studying endlessly, EduRev has designed CA Foundation study material, with Structured Courses, Videos ... 於 edurev.in -
#65.Codeforces Contest 1117 problem C Magic Ship——二分
... all positions in the sea can be described by cartesian plane) and you want to travel to a point (x2,y2).You know the... 於 blog.csdn.net -
#66.Solving Equations with e and lnx
Solve for x (hint: put u = ex, solve first for u):. 4. ex + e−x ex - e−x ... e2x · eln x. (y + 1) · (y - 1) = e2x · x y2 - 1 = xe2x y2. = xe2x + 1. 於 www.mit.edu -
#67.n2y: Special Education Software, Curriculum & Learning Tools
Achieve Compliance with Confidence See how you can achieve and maintain compliance while boosting student performance! Book a Demo. Why n2y? Because we believe ... 於 www.n2y.com -
#68.Slope Of 3 4 - bei Carmela Cosmetic
How do you find the slope of (3,4) (4,6)? Algebra Graphs of Linear Equations and ... Formula: m = (y2 - y1) / (x2 - x1) Example: Find the slope for ... 於 carmelacosmetic.de -
#69.16.6 Parametric Surfaces and their Areas (u, v) ∈ D v u D (u, v) r
y2. Definition. The grid lines of a parametric surface S are the two families of curves for which either u or v is constant. 於 www.math.uci.edu -
#70.Y2 - "Beast Mode" (Official Audio) - YouTube
0:00 · New! Watch ads now so you can enjoy fewer interruptions. Got it ... 於 www.youtube.com -
#71.2 Complex Functions and the Cauchy-Riemann Equations
5. f(z) = z2, with F(x, y)=(x2 − y2) + i(2xy) (u = x2 − y2, ... 於 www.math.columbia.edu -
#72.You are given the circle x2+y2+ax+by+c=0 , where a
You are given the circle x2+y2+ax+by+c=0 , where a, b and c are some constants. Given that this circle passes through the points 4,2,0,3 and 3,-2 . 於 www.gauthmath.com -
#73.Can you move your feet to get green streets? (Class 8 Road ...
Y2 : 2018.19 Learning exploration – Can you move your feet to get green streets? (Class 8 Road safety). Autism support for parents. 於 greentopschool.co.uk -
#74.Parametric Surfaces and Surface Area - Purdue Math
x2 + y2 = R2 sin2(u), and therefore x2 + y2 + z2 = R2. That tells us that our surface is subset of the sphere centered at the origin. Using the domain. 於 www.math.purdue.edu -
#75.You 2: A High Velocity Formula for Multiplying Your Personal ...
Amazon.com: You 2: A High Velocity Formula for Multiplying Your Personal Effectiveness in Quantum Leaps: 8601405167934: Price Pritchett: Books. 於 www.amazon.com -
#76.1pm pdt
1pm pdt. Join game designer Tomas and Doug live from GAMA Expo tomrrow at 1PM PDT/4PM EDT/10PM CET. They promise to update you on upcoming games & be sure ... 於 iris-markt.de -
#77.Primary assessments: future dates - GOV.UK
The key stage 1 tests should be administered during May 2023. ... To add a full list of all important key stage 1 dates to your calendar, you can download the Key ... 於 www.gov.uk -
#78.ordinary differential equations - Find the value of $W(y_1,y_2)(5)
You are right in that you need to rewrite the equation so that y′′ ... on the Wronskian, and not on the separate values of y1(t) and y2(t). 於 math.stackexchange.com -
#79.Examples of changing the order of integration in double ...
If you integrate with respect to x first, you will obtain an integral that ... There is no antiderivative of ey2, so you get stuck trying to compute the ... 於 mathinsight.org -
#80.Y2 - YouTube
0:00 · New! Watch ads now so you can enjoy fewer interruptions. Got it ... 於 www.youtube.com -
#81.Program to calculate distance between two points
You are given two coordinates (x1, y1) and (x2, y2) of a two-dimensional graph. Find the distance between them. Examples:. 於 www.geeksforgeeks.org -
#82.Y2 Yoga - Charlotte's Leading Hot Yoga Studio
Y2 Yoga offers THREE state-of-the-art yoga studios with great amenities, ... Just Flow with It. At Y2 Yoga, you might find our approach to yoga a bit out of ... 於 y2yoga.com -
#83.Y2 Chute | Freeride Skiing route in Colorado - FATMAP
Once you ski pass the low gradient pine forest, you will come to another bald aspect with multiple gulches that descend into the Y2 drainage. These 4-5 micro- ... 於 fatmap.com -
#84.Optimization with Constraints The Lagrange Multiplier Method
production possibility frontier 200 = x2 + y2, and she consumes all the goods herself. Her utility function is u = x О y3. FInd her utility maximizing x and ... 於 www.sfu.ca -
#85.16.9 Change of Variables in Multiple Integrals Recall
Recall: For sinlge variable, we change variables x to u in an integral by the formula. (substitution rule) ... (a) R is the ellipse x2 + y2. 於 www3.nd.edu -
#86.Redmi Y2 (Blue, 32 GB) (3 GB RAM) - Flipkart
Its powerful Qualcomm Snapdragon 625 processor and 3 GB of RAM help you use all its innovative features without any lag. Click beautiful pictures and watch ... 於 www.flipkart.com -
#87.Level surfaces
Now let's step up a dimension and consider functions w=f(x,y,z):U⊆R3→R of 3 variables; one such function is w = f(x,y,z) = x2+y2−z2. 於 web.ma.utexas.edu -
#88.How do you plot horizontal bar charts with x1, y1 and x2, y2 ...
Since you seem to be a new gnuplot user (but not a new StackOverflow member, as such you should now that some code is expected), ... 於 stackoverflow.com -
#89.Implicit Differentiation - Math is Fun
You may like to read Introduction to Derivatives and Derivative Rules first. ... Use the Chain Rule (explained below): d dx (y2 ) = 2y dy dx. 於 www.mathsisfun.com -
#90.What does Y2 mean in Internet?You Too - Online Abbreviations
Y2 is an acronym which stands for "You Too". This phrase is a colloquialism used as a polite way to say goodbye to someone. In this context, the phrase ... 於 www.onlineabbreviations.com -
#91.Quadratic Simultaneous Equations - Maths Genie
Read each question carefully before you start to answer it. •. Keep an eye on the time. ... y=2-3xc. 2 x² + (2 - 3x)². 20 x² +(2-3x) (2-3x) = 20 x² + 4-6x. 於 www.mathsgenie.co.uk -
#92.Solved You are given the circle, x2 + y2- 25 (a) At what two
Question: You are given the circle, x2 + y2- 25 (a) At what two points is x = 4? Point A: (4,-3 (smaller y value) (larger y value) (b) Find the equation of the ... 於 www.chegg.com -
#93.If y=1/(x^x), how do you show that y2(1) =0? - Quora
If “y2” is the second derivative then so that . Differentiating both sides with respect to x,. To find the second derivative, differentiate ... 於 www.quora.com -
#94.Y2 Residence Hotel in Makati City | Official Hotel Website
Y2 Residence Hotel provides a serene and tranquil ambiance, spacious hotel rooms, and excellent customer service. ... EVERYTHING YOU NEED TO RELAX. Y2 ... 於 www.y2hotel.com -
#95.Laplace's equation in the Polar Coordinate System
As I mentioned in my lecture, if you want to solve a partial differential equa- ... ∂y2. = uxx +uyy = 0. (1). The Cartesian coordinates can be represented ... 於 www.math.ucdavis.edu -
#96.Distance Formula - Expii
The Distance Formula can be used to find the distance between two points on the coordinate plane. Let's say you have any two points, (x1,y1) and (x2,y2), and ... 於 www.expii.com -
#97.Solutions ∗ Introduction to multiple integrals - math.illinois.edu
(Hint: When you change to dxdy, be sure to also change the bounds of ... Consider the region bounded by the curves determined by −2x + y2 = 6 and −x + y ... 於 faculty.math.illinois.edu -
#98.State-Trait-Anxiety-Inventory.pdf
STAI Form Y-1 and Form Y-2 ... You have purchased permission to reproduce this document up to the maximum number that is shown on. 於 oml.eular.org -
#99.SOLUTIONS Problem 1. Find the critical points of the function f ...
the line y = 2: ... x = u. √ v, y = 1 uv2 . Using the chain rule, compute the derivatives. ∂w. ∂u ... f(x, y) = sin(x2 + y2) ln(x4y4 + 1) tan(xy). 於 math.ucsd.edu