loop的問題,我們搜遍了碩博士論文和台灣出版的書籍,推薦Bayron, Kalynn寫的 My Dear Henry: A Jekyll & Hyde Remix 和的 Ssa-Based Compiler Design都 可以從中找到所需的評價。
這兩本書分別來自 和所出版 。
明新科技大學 電機工程系碩士班 蘇信銘所指導 黃禎岳的 無橋式功因修正轉換器研製 (2021),提出 loop關鍵因素是什麼,來自於功率因數修正器、平均電流控制法、圖騰柱型功率因數修正器。
而第二篇論文國立臺北科技大學 電子工程系 曾柏軒所指導 林聖曄的 考量CSI相位偏移偵測與校正之室內定位演算法 (2021),提出因為有 深度學習、通道狀態資訊、相位偏移、訊號強度、室內定位的重點而找出了 loop的解答。
My Dear Henry: A Jekyll & Hyde Remix
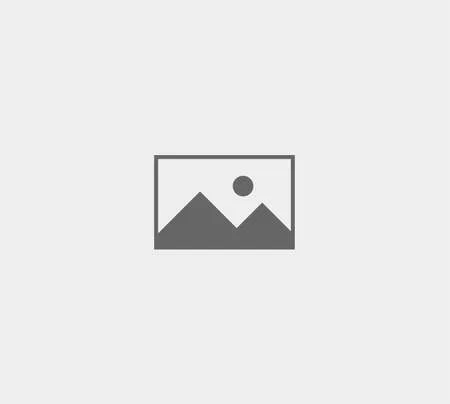
為了解決 loop 的問題,作者Bayron, Kalynn 這樣論述:
Kalynn Bayron is the bestselling author of Cinderella Is Dead, This Poison Heart and My Dear Henry: A Jekyll & Hyde Remix. She is a classically trained vocalist and when she’s not writing you can find her listening to Ella Fitzgerald on loop, attending the theater, watching scary movies, and spendin
g time with her kids. She currently lives in upstate New York with her family.
loop進入發燒排行的影片
無橋式功因修正轉換器研製
為了解決 loop 的問題,作者黃禎岳 這樣論述:
本論文目的在研製一無橋式功因修正轉換器,硬體電路以圖騰柱型功率因數修正電路為核心,利用外迴路電壓感測電路與內迴路電流感測電路完成本控制。本研究採用平均電流控制法來實現功率因數修正功能。平均電流控制法以雙迴圈PI控制器來實現,由輸入電壓極性與波形角度傳給雙迴圈PI控制系統運算,外迴圈PI控制器控制電壓,內迴圈PI控制器控制電流,軟體是以瑞薩電子公司生產的R5F562TAADFP數位訊號處理器實現,經實測結果顯示功率因數可達0.98以上,總諧波失真率最大為11.644%。證明本控制器可達功率因數修正的效果。
Ssa-Based Compiler Design
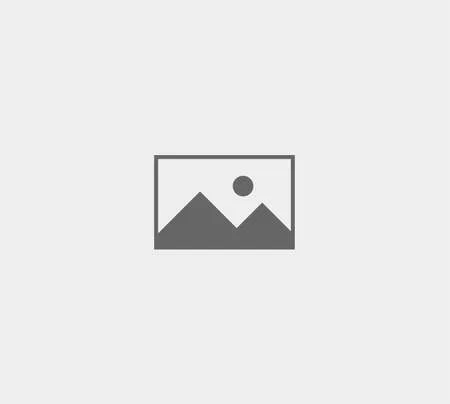
為了解決 loop 的問題,作者 這樣論述:
Fabrice Rastello is an Inria research director and the leader of the CORSE (Compiler Optimization and Runtime SystEms) Inria team. His expertize includes automatic parallelization (PhD thesis on tiling as a loop transformations), and compiler back-end optimizations (engineer at STMicroelectronics’s
compiler group + researcher at Inria). Among others, he advised several PhD thesis so as to fully revisit register allocation for JIT compilation in the light of Static Single Assignment (SSA) properties. He likes mixing theory (mostly graphs, algorithmic, and algebra) and practice (industrial trans
fer). His current research topics include: (i) combining run-time techniques with static compilation, hybrid compilation being an example of such approach he is trying to promote; (ii) performance debugging through static and dynamic (binary instrumentation) analysis; (iii) revisiting compilers infr
astructure for pattern specific programs.Florent Bouchez Tichadou received his Ph.D. in computer science in 2009 at the ENS Lyon in France, working on program compilation. He was then a post-doctoral fellow at the Indian Institute of Science (IISc) in Bangalore, India. He worked for three years at K
alray, a startup company in the Grenoble area in France. Since 2013, he is an assistant professor at the Université Grenoble Alpes (UGA).
考量CSI相位偏移偵測與校正之室內定位演算法
為了解決 loop 的問題,作者林聖曄 這樣論述:
通道狀態資訊(Channel StateInformation, CSI)可用於室內定位,起到監視人們生活的作用。它使用Wi-Fi多通道訊號,不受光源、聲音干擾,並具備優異的角度、距離感測能力。本文研究中心頻率5.22GHz,頻寬20MHz,56子載波的CSI量測值。在9個不同位置,收集實驗室中57個位置傳送的CSI訊號。在本研究中,我們發現隨機π跳動問題,使得每根天線的相位可能出現±π偏移,這主要是硬件的鎖相環造成的。由於相位的不同,三根天線之間有四種可能的相位差組合。為了估計使用者的位置,我們把CSI量測值轉化為熱力圖作為深度學習網路模型的輸入,來解決本問題。為了克服多路徑效應,經由多訊
號分類(Multiple Signal Classification, MUSIC)計算出到達角(Angle of Arrival, AoA)與飛行時間(Time of Flight, ToF)的熱力圖。然而,由於ToF量測平台存在延時偏移,在本研究中,把熱力圖最大值對應的距離平移到信號強度(Received Signal Strength Indicator, RSSI)對應的距離,再以接入點(access point, AP)的位置為中心,朝向為AoA參考方向,把極坐標轉為直角坐標。由於每根天線可能有π相位偏移,三根天線之間有四種相位組合,所以每筆資料的Rx有四張熱力圖。本文以卷積神經網路
(Convolutional Neural Network, CNN)、殘差神經網路(Residual Neural Network, ResNet)等神經網絡組成的深度學習網路(Deep Learning based wireless localization, DLoc),用訓練出的模型對不同位置的預測準確度,來探究AP數量、相位校正等因素對深度學習效能的影響,並與深度卷積網路(Deep Neural Network, DNN)和SpotFi的方法在校正π相位偏移的效能上作對比。