In case of need mean的問題,透過圖書和論文來找解法和答案更準確安心。 我們找到下列問答集和資訊懶人包
In case of need mean的問題,我們搜遍了碩博士論文和台灣出版的書籍,推薦寫的 The Year in Tech, 2023: The Insights You Need from Harvard Business Review 和Gano, Elisa的 Truly Broken都 可以從中找到所需的評價。
這兩本書分別來自 和所出版 。
國立中正大學 資訊管理系研究所 胡雅涵、李珮如所指導 宋昇峯的 以監督式機器學習探討電子病歷中非結構化資料對早期預測中風後功能復原後果之價值 (2021),提出In case of need mean關鍵因素是什麼,來自於急性缺血性中風、電子病歷、功能復原後果、機器學習、敘述式臨床紀錄、自然語言處理、風險模型、預測。
而第二篇論文國立臺灣科技大學 資訊工程系 范欽雄所指導 莊智翔的 使用預訓練語言模型進行法律案例檢索之研究 (2021),提出因為有 案例檢索、預訓練語言模型、深度學習、文件重排序、Transformer的重點而找出了 In case of need mean的解答。
The Year in Tech, 2023: The Insights You Need from Harvard Business Review
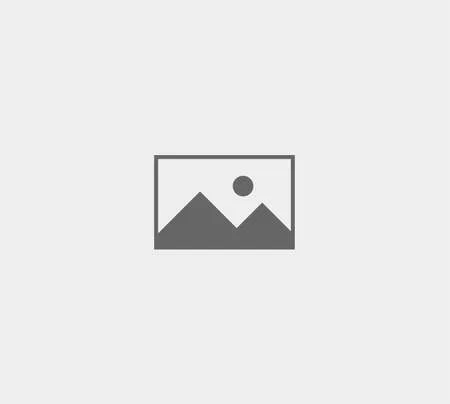
為了解決In case of need mean 的問題,作者 這樣論述:
A year of HBR’s essential thinking on tech--all in one place.Easy-to-use AI tools, contactless commerce, crypto for business, the mature metaverse--new technologies like these are reshaping organizations at the hybrid office, on factory floors, and in the C-suite. What should you and your company
be doing now to take advantage of the new opportunities these technologies are creating--and avoid falling victim to disruption? The Year in Tech 2023: The Insights You Need from Harvard Business Review will help you understand what the latest and most important tech innovations mean for your organ
ization and how you can use them to compete and win in today’s turbulent business environment.Business is changing. Will you adapt or be left behind?Get up to speed and deepen your understanding of the topics that are shaping your company’s future with the Insights You Need from Harvard Business Rev
iew series. Featuring HBR’s smartest thinking on fast-moving issues--blockchain, cybersecurity, AI, and more--each book provides the foundational introduction and practical case studies your organization needs to compete today and collects the best research, interviews, and analysis to get it ready
for tomorrow.You can’t afford to ignore how these issues will transform the landscape of business and society. The Insights You Need series will help you grasp these critical ideas--and prepare you and your company for the future.
In case of need mean進入發燒排行的影片
One Of The Secrets To Why You Don’t Need A High Paying Job To Become A Millionaire Is By Having Money Working For You. If You Want All Of Dan’s Investing Secrets To Do That, Click Here To Get Your Ticket Today: http://nohighincomejob.danlok.link
Contrary to popular belief, you don’t need a high paying job to become a millionaire. Now, you may be thinking the obvious answer is by starting a business. But that’s rarely the case! So in today’s video, Dan Lok is going to share why you don’t need a high paying job to become a millionaire, and what to do instead.
? SUBSCRIBE TO DAN'S YOUTUBE CHANNEL NOW ?
https://www.youtube.com/danlok?sub_confirmation=1
Check out these Top Trending Playlists -
1.) Boss In The Bentley - https://www.youtube.com/playlist?list=PLEmTTOfet46OWsrbWGPnPW8mvDtjge_6-
2.) Sales Tips That Get People To Buy - https://www.youtube.com/watch?v=E6Csz_hvXzw&list=PLEmTTOfet46PvAsPpWByNgUWZ5dLJd_I4
3.) Dan Lok’s Best Secrets - https://www.youtube.com/watch?v=FZNmFJUuTRs&list=PLEmTTOfet46N3NIYsBQ9wku8UBNhtT9QQ
Dan Lok is a Chinese-Canadian business magnate and global educator known for being the founder and chairman of Closers.com - the world’s #1 virtual-closers network, Copywriters.com, and SalesCalls.com. Beyond his businesses, Mr. Lok has led several global movements to redefine modern education where he has taught individuals from 150+ countries to develop high income skills and financial confidence.
Beyond his success in business, he was also a two time TEDx opening speaker. An international best-selling author of 12+ books. A member of Young Presidents Organization (YPO) - a private group of global chief executives whose companies employ 22 million people and generate 9-trillion USD in annual revenues. He also hosts The Dan Lok Show - a series on elite business tycoons and world-leading entrepreneurs.
Today, Mr. Lok continues to be featured in thousands of media channels and publications every year and is widely seen as one of the top business leaders by millions around the world.
If you want the no b.s. way to master your financial destiny, then learn from Dan. Subscribe to his channel now.
★☆★ CONNECT WITH DAN ON SOCIAL MEDIA ★☆★
YouTube: http://youtube.danlok.link
Dan Lok Blog: http://blog.danlok.link
Facebook: http://facebook.danlok.link
Instagram: http://instagram.danlok.link
Linkedin: http://mylinkedin.danlok.link
Podcast: http://thedanlokshow.danlok.link
#DanLok #HighIncomeJob #Millionaire
Please understand that by watching Dan’s videos or enrolling in his programs does not mean you’ll get results close to what he’s been able to do (or do anything for that matter).
He’s been in business for over 20 years and his results are not typical.
Most people who watch his videos or enroll in his programs get the “how to” but never take action with the information. Dan is only sharing what has worked for him and his students.
Your results are dependent on many factors… including but not limited to your ability to work hard, commit yourself, and do whatever it takes.
Entering any business is going to involve a level of risk as well as massive commitment and action. If you're not willing to accept that, please DO NOT WATCH DAN’S VIDEOS OR SIGN UP FOR ONE OF HIS PROGRAMS.
This video is about Why You Don't Need A High Income Job To Become A Millionaire
https://youtu.be/VRMnLcgYVTA
https://youtu.be/VRMnLcgYVTA
以監督式機器學習探討電子病歷中非結構化資料對早期預測中風後功能復原後果之價值
為了解決In case of need mean 的問題,作者宋昇峯 這樣論述:
中風是導致成人殘障的重要原因,中風功能復原後果的精準預測,能協助病人及家屬及早準備後續照顧事宜,衛生政策制定者也能依此預測結果適切規劃人力與資源,以投入中風病人的急性後期與中長期照護。目前的中風功能復原後果預測模型皆是以結構化資料建立,甚至最新使用數據驅動方式發展的機器學習預測模型依然是以結構化資料為主。相對的,照顧病人所製作的大量敘述式病歷文字紀錄,即非結構化資料,反而甚少被使用。因此,本研究的目的,即是使用監督式機器學習來探討非結構化臨床文字紀錄於急性缺血性中風後之初期預測功能復原後果之應用價值。在6176位2007年10月至2019年12月間因急性缺血性中風住院之病人中,共3847位病
人符合本研究之收案/排除條件。我們使用自然語言處理,萃取出住院初期之醫師紀錄及放射報告中之臨床文字紀錄,並且實驗了不同文字模型與機器學習演算法之組合,來建構中風功能復原後果的預測模型。實驗發現使用醫師紀錄時,操作特徵曲線下面積為0.782至0.805,而使用放射報告時,曲線下面積為0.718至0.730。使用醫師紀錄時,最好的組合為詞頻-倒文件頻加上羅吉斯迴歸,而使用放射報告時,最好之組合為基于轉換器的雙向編碼器表示技術加上支持向量機。這些基於純文字的機器學習預測模型並無法勝過傳統的風險模型,這些傳統模型的曲線下面積為0.811至0.841。然而,不管是以曲線下面積、重分類淨改善指標、或整合式
區辨改善指標來評估,臨床文字紀錄中的資訊的確可以增強傳統風險模型的預測效能。本研究之結論為,電子病歷中的非結構化文字經過自然語言處理後,不僅可以成為另類預測中風功能復原後果的工具,更可以增強傳統風險模型的預測效能。透過演算法來自動擷取並整合分析結構化與非結構化資料,將能提供醫師更好的決策支援。
Truly Broken
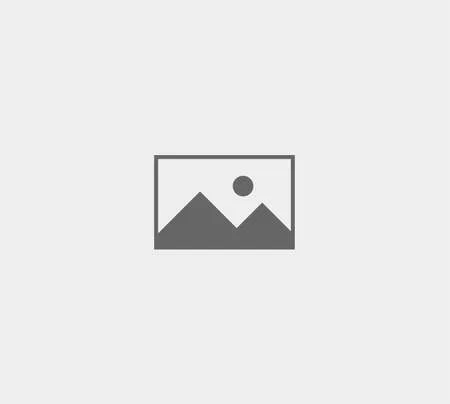
為了解決In case of need mean 的問題,作者Gano, Elisa 這樣論述:
Does anyone ever stop and think what they’re about to do? How their action can affect people’s lives? I heard once or twice that your decisions are like a domino effect, one decision can affect many. Why make choices that you know you will regret? Why put people through pain? Can you actually say th
at you ever thought about how many people you hurt? Explain in so many words why we treat people like they don’t mean anything. Many of us are the victims in cases like these. Maybe you are the victimizer, in that case describe why you felt that treating someone so bad would make you feel better? We
ll, did it? Maybe you should look in the mirror and ask if you are truly happy with being yourself? Or maybe you feel the need to take out all the pain you bottle up on someone who doesn’t deserve it. Maybe it’s just me but I am done with thinking of all the reasons why people get involved in situat
ions they can’t dig themselves out of, well here is my story...
使用預訓練語言模型進行法律案例檢索之研究
為了解決In case of need mean 的問題,作者莊智翔 這樣論述:
法律判決運行了許多世紀,在當今社會所有的案例判決都會產生法律判決書,而其產生龐大的資料,使得法律從業人員在查閱上需要大量的時間,雖然現今都已將案例資料數位化,但在數位化後如何幫助法律從業人員快速地找到其想要查詢的案例,成為重要的議題。近年來,深度學習技術應用於法律領域方面,大部分都關注在案例分類以及判刑預測上,而在案例檢索多半使用傳統的檢索方法,本論文將自然語言處理技術應用在案例檢索上,我們分析法律案例並將它映射到向量空間中,並透過歷年的法律案例建立語義模型,找出與查詢案例相關的關聯案例,使法律從業人員能夠快速地掌握相關案例,針對法律案例檢索系統我們提出了兩種基於深度學習的自動化檢索方法,分
別是基於文件長度的模型與基於段落長度的模型,這兩種方法主要使用Transformer的變體模型,並且檢索流程都可以被分為兩個階段,分別是檢索和重新排名。本論文以COLIEE-2020競賽的任務1資料集進行研究及實驗,任務1的競賽目標是找到一個可靠且穩定的法律案例檢索系統,我們使用召回率及F1分數評估模型在COLIEE-2020競賽的效果,由於我們的兩種方法都被分為兩階段,在第一階段我們會以召回率當作評估方法,我們提出的基於段落長度的模型召回率達93.87%,相較於實驗中其他檢索方法,我們的基於段落長度模型具有最高的召回率;第二階段我們會使用F1分數來評估模型,我們提出的基於段落長度模型F1分數
達到61.2%,相比於其他常見的檢索方法,我們具有最好的效果。